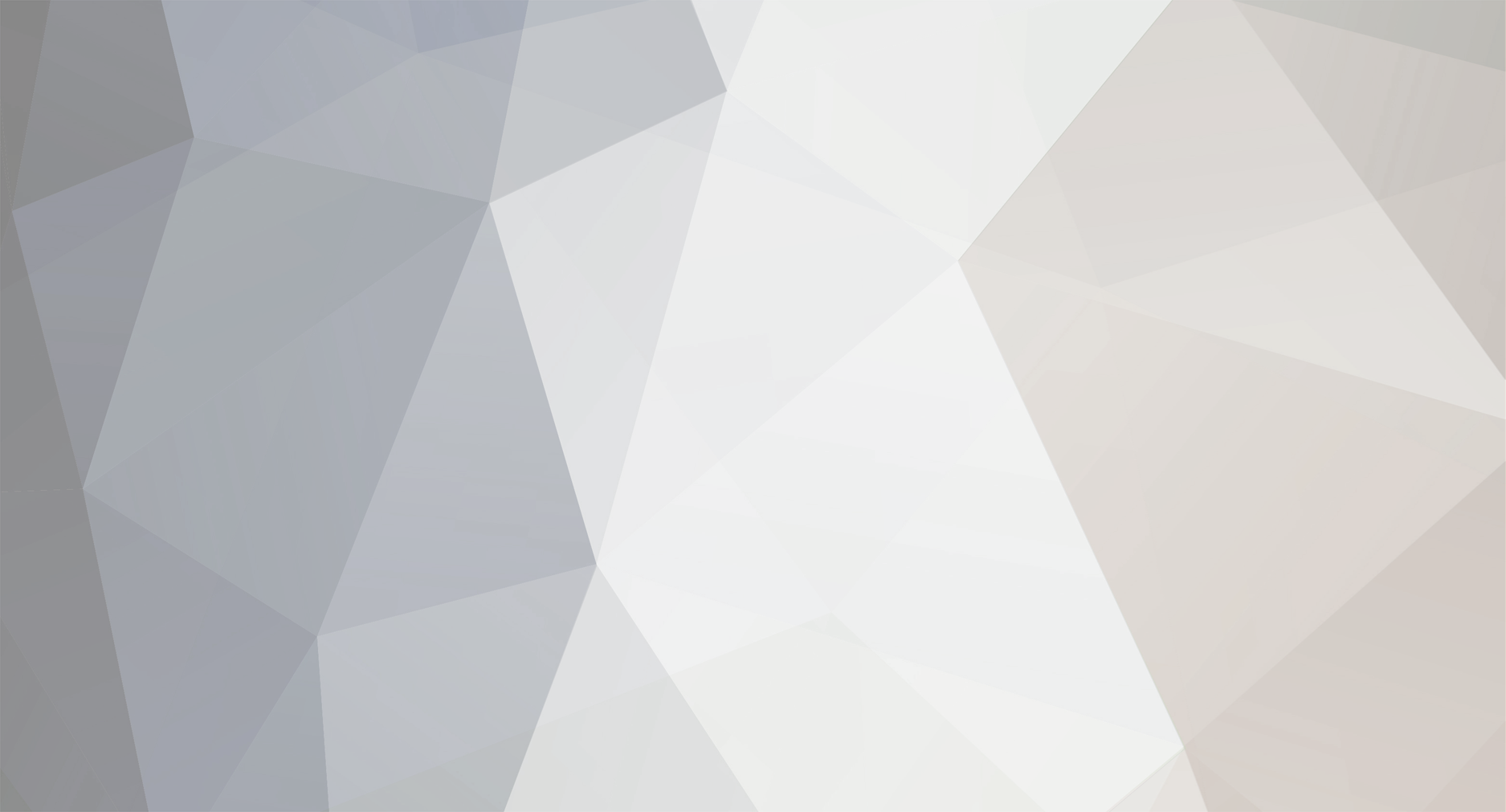
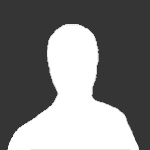
Sarahisme
Senior Members-
Posts
826 -
Joined
-
Last visited
Content Type
Profiles
Forums
Events
Everything posted by Sarahisme
-
is that like the 95% confidence interval thing? should i do something such as giving the mean and the 95% confidence level?
-
anyone? lol, or is thermodynamics not a favourite around here
-
nevermind, i'll just graph it and take the 3 lines of bestfit. speaking of which, does anyone know a program which will give you the error in your line of best fit?
-
hey heres the question & heres my answer, but i got stuck so yep any help would be greatly apprectiated! ok here is what i have got so far... [math] \epsilon = 1 - \frac{Q_{out}}{Q_{in}} [/math] [math] Q_{out} \ = \ |Q_{d->a}| \ = \ C_v|T_a - T_d| \ = \ C_v(T_d - T_a) [/math] [math] Q_{in} \ = \ Q_{b->c} = C_p(T_c - T_b) [/math] so [math] \epsilon \ = \ 1 - \frac{C_v(T_d - T_a)}{C_p(T_c - T_b)} [/math] but [math] \frac{C_p}{C_y} \ = \ \gamma [/math] So [math] \epsilon = 1 - \frac{T_d - T_a}{ \gamma (T_c - T_b)} [/math] then using PV = nRT [math] \epsilon = 1 + \frac{1}{ \gamma} \frac{P_aV_a - P_dV_d}{P_cV_c - P_bV_b} [/math] now using the fact that [math] V_a = V_d [/math] and [math] P_c=P_b [/math] [math] \epsilon = 1+ \frac{1}{ \gamma} \frac{V_a(P_a - P_d)}{P_c(V_c-V_b)} [/math] now dividing top and bottom by [math] V_aP_c [/math] [math] \epsilon = 1 + \frac{1}{ \gamma} \frac{\frac{P_a}{P_c} - \frac{P_d}{P_c}}{ \frac{V_c}{V_a} - \frac{V_b}{V_a}} [/math] now [math] PV^{ \gamma } = constant[/math] so [math] P_cV_c^{ \gamma } = P_dV_d^{ \gamma } [/math] then [math] \frac{P_d}{P_c} = \frac{V_c^{ \gamma }}{V_d^{ \gamma }} = ( \frac{V_c}{V_d} )^{ \gamma } [/math] and similarly [math] \frac{P_a}{P_b} = \frac{V_b^{ \gamma }}{V_a^{ \gamma }} = ( \frac{V_b}{V_a} )^{ \gamma } [/math] so [math] \epsilon = 1 + \frac{1}{ \gamma} \frac{( \frac{V_b}{V_a} )^{ \gamma } - ( \frac{V_c}{V_d} )^{ \gamma }}{ \frac{V_c}{V_a} - \frac{V_b}{V_a}} [/math] this is where i get stuck, any suggestions guys n' gals? Sarah
-
ok, but how can the average error be less than all the individual errors? also, what do you guys think of method of getting [math] \pm 6 [/math]
-
hey i am writing up my prac report i have a question about error caluclation. if i take the average of serveral measurements (instead of drawing a graph), and these measurements are [math] 147 \pm 3 [/math] [math] 146 \pm 3 [/math] [math] 143 \pm 3 [/math] [math] 145 \pm 3 [/math] [math] 146 \pm 2 [/math] i get ther average to be 145 (to 3 sig. figs.) , now how do i calucalte the error? do i use a 95% confiddence interval or somethign else? I have on method i think might work, but it gives a very big error compared to the ones for the orginal measurements: this is the method: [math] ( \delta avg)^{2} = ( \delta m_1)^{2} + ( \delta m_2)^{2} + ( \delta m_3)^{2} + ( \delta m_4)^{2} + ( \delta m_5)^{2} [/math] where [math] m_1 , m_2, ... [/math] are measurement 1, measurement 2,... so [math] (\delta avg) = \sqrt(3^{2} + 3^{2} + 3^{2} + 3^{2} + 2^{2}) = \sqrt(40) = 6 [/math] so avg = [math] 145 \pm 6 [/math] but this error is quite large compared to the others so yeah... any advice would be great! Thanks guys _Sarah
-
oh ok, yep thanks for that
-
yeah its cool, i got it in the end, you do it by trying to get lineraly dependent set sort of thing thanks for trying though
-
ok heres my attempt at what i think the proof to problem should look like (tell me what you think! ) ok here goes: ----------------------------------------------------------------------------------------- ----------------------------------------------------------------------------------------- Define T to be [math] T( \sum_{i=1}^n(a_iv_i)) = \sum_{i=1}^n(a_iw_i) [/math] Since T is defined as transforming the basis vectors of the vector space V, then it is well defined. Now take [math] v, p \in V [/math] and [math] c,d \in \mathbb{R} [/math] (i.e. c,d scalars) So [math] v = \sum_{i=1}^n(a_iv_i) [/math] [math] p = \sum_{i=1}^n(b_iv_i) [/math] [math] (cv + cp) = c(\sum_{i=1}^n(a_iv_i)) + d(\sum_{i=1}^n(b_iv_i)) [/math] [math] = v_i(\sum_{i=1}^n(ca_i + db_i)) [/math] [math] = \sum_{i=1}^n(v_i(ca_i + db_i)) [/math] Then [math] T(cv + dp) = \sum_{i=1}^n(ca_i + db_i)w_i [/math] [math] = c\sum_{i=1}^na_iw_i + d\sum_{i=1}^nb_iw_i [/math] [math] = cT(v) + dT(p) [/math] [math] \therefore [/math] T is linear. [math] \therefore [/math] there is a unique linaer transformation [math] T : V \rightarrow W [/math] such that [math] T(v_i) = w_i [/math] for i = 1,…,n. ----------------------------------------------------------------------------------------- ----------------------------------------------------------------------------------------- well that’s what I think it is, however I am not sure this shows that it is unique (or is it because of the fact that is transforms basis vectors that make it unique??, in which case should I say up the top of my proof “Since T is defined as transforming the basis vectors of the vector space V, then it is well defined AND unique.”) well anyway, tell me what you think! Cheers Sarah
-
So to do this problem do i need to know stuff about difference equations ?? (its a chapter in my linear algebra book) ??
-
oh ok i think i see.... a linear transformation maps one vector space into another, right? so if T:E --> W is a linear transformation the range of the transformation is depedent on the vectors in V, and if you have a basis for E, then you effectively have all basis for the range of T, as a linear transformation is defined by T(u+v) = T(u) + T(v) and T(cu) = cT(u) is what i just said correct?....or relevant? Thanks Sarah
-
"a liner map is uniquely determined by where it sends a basis and specifying the action on the basis uniquely determines the map obviously" is that a thm. of some kind, because i havent seen it in my textbook yet. although i can see that it makes sense.
-
nevermind, i got it! -Sarah
-
hmmm....yeah i dunno, i can't find anything in my text book that seems to help me, there is stuff about linear transformations, but sort of dunno i don't see how to apply it to this particular question :S
-
ok, so now i am thinkning that it has something to do with matrices and linear transformations..... is that the right line of thought?
-
dammit i wish i knew which damn angle they were talking about!! (i think its the one between the leftmost parrallel line from the virtual image and the axis line????¿¿¿¿¿??????)
-
umm i think i've got the proof, i'll be back in a few hours, so i'll put it up then
-
i am not even sure which angle they are talking about on the diagram. :S
-
ok, cool! , yep i think i got it right this time too (i've checked though it a few times, and it makes sense, and still gives me the same answer each time )
-
ok umm so how about i start by well... lol i am not really sure how to start a problem like this, never really done one like it before. how can you show that there is a unique linear transformation? i dunno :S
-
i think it was this part of my orginal answer that was wrong: we get [math] 1-\frac{v^2}{c^2} = 0.67 [/math] oh and this bit of it too so [math] m = kinetic mass = \frac{E}{c^2} = 8.224\times10^{-28} kg [/math] i guess i just must have been pressing the wrong buttons on my calculator!