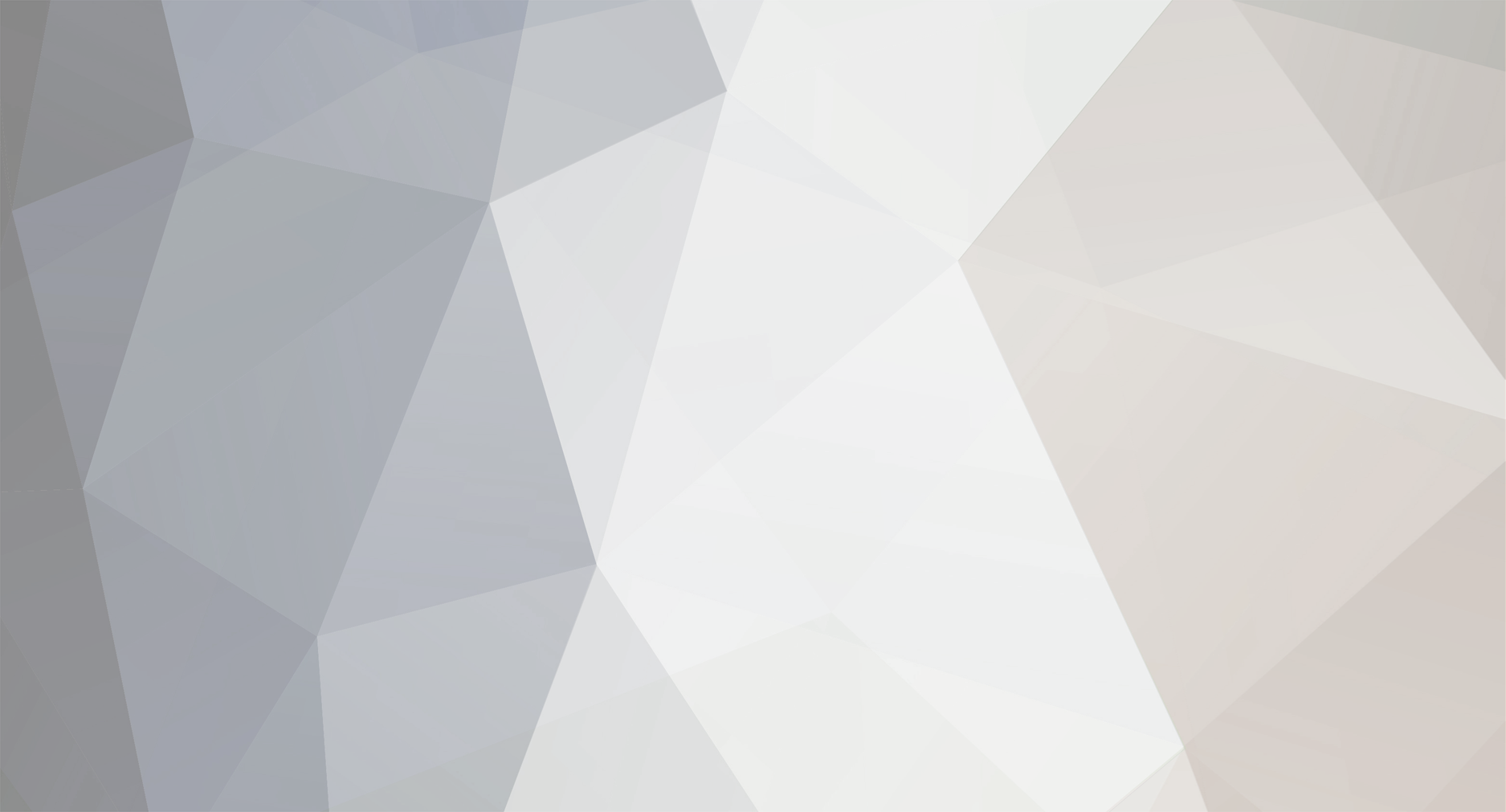
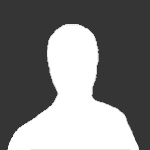
nonstoptaxi
Members-
Posts
11 -
Joined
-
Last visited
Retained
- Quark
nonstoptaxi's Achievements

Quark (2/13)
10
Reputation
-
Hi all, can someone supply advice on this: I am determined to analyse a 3 way interaction of variables a x b x c. No problem there. But since I'm using hierarchical multiple regression, I am following aiken and west's steps of centering my data to produce interaction terms, which is again fine. My query lies in producing the graphs to understand the signficant 3 way interaction that has been found. I can (just about) easily do a 2 way interaction and represent that graphically. However, the problem is the way i do a 3 way interaction. Basically, I'm going to use the median split method of separating my sample on the 3rd predictor © to produce a high and low grouping. Then I'm going to rerun my analysis, having computed NEW centered variables based on the changes in the mean for variable c, again on the two separate groups using the initial hiearchical steps of the regression with main and interaction terms. Finally, I can produce two 2-way interaction graphs, one of high and one of low on predictor c. Though I've seen this method done before (seen in research published in a journal), this is my question: If the initial full set of data produced a significant 3 way interaction, won't splitting the data into two and then rerunning the analysis (as noted above) remove the signficance? And therefore doing the graphs will come out with rubbish? thanks for your help. J
-
Hi Glider and others, in terms of conducting power analysis for one test, fair enough. But to your knowledge, can you conduct a power analysis for a correlational test? If yes, what are the options for looking at what level of 'r' you would count as substantive when you get a number of zero-order corrleations? As in, where do you draw the line and say 'I'll just consider those rs above a certain level'? If this is so, is there a reference for this as well? Any advice greatly received Jon
-
Glider, that makes sense. I guess as someone once said, stats can be our best friends or our worst enemies (or was that computers....or the tele?). I'm glad it's a matter of making a common sense judgement a priori, rather than conducting some bizarre statistical test thanks again! J
-
Thanks for the responses Glider and 5614. Glider, thanks for your indepth response. U always come through on these stats questions! You nearly make stats palatable! U must be some psych. stats lecturer. Yeah, I'm aware of power analysis, and I think 'g-power' is the free program circulating around my department, which I should really learn to use. But in terms of specifying the effect size...how does one do that? I know how to calculate an effect size post hoc for a t-test for instance. But how do I do it in advance? As for experimental power, it's 0.8 'cause Cohen said or something, and alpha is usually set at .05. So it's just effect size that is confusing me. Thanks. John
-
Hi all, can anyone tell me the lowest sample size needed for an experiment? Coolican (Research Methods and Statistics in Psychology) suggests 25-30 as the lowest number. So does this mean having 25 in each condition, or 25 as the overall size? I ask because I 19 in condition A, 100 in the other). I was unable to get a matched sample size. Please advice if at all possible. Thanks,
-
Hi everyone, can someone give me advice on sample size when conducting a linear regression using the backward method of entry (note the backward entry, as this has created this quandry)? I've seen various guidelines about sample size in relation to the number of IVs being investigated (e.g., Tabachnick & Fidell) who suggest N >= 104 + m, where m = number of independent variables. If this is the case, do I calculate my sample size: a) of 104 and the value of 'm' after running the multiple regression (strange I know), so that I can see the number of predictors appearing in the final model after all non-significant predictors have been discarded? b) Or is the inverse correct, whereby I calculate 104 plus the value of 'm', where 'm' is taken to be the number of IVs inputted into the multiple regression to begin with? I basically need to know since there are cases of missing data, and I'd much rather exclude them listwise, but the final N hovers around 110 (fine if sample size is calculated via method (a) above since the final model produced by the multiple regression has about 6 predictors), but obviously not fine when considering (b) as I have about 20 IVs inputted into the model at the start. I've also noted guidance where sample size should be 20:1 (20 cases for every one IV) - again, problematic if calculated with (b), but not (a). Hope that makes sense to someone out there. Here's hoping and praying for guidance. Thanks all. Jon
-
statistics question - para. vs non-para.
nonstoptaxi replied to nonstoptaxi's topic in Psychiatry and Psychology
Thanks guys. Glider, yes, that's an interesting response. I'm a postgrad...so yay, I can be lazy! Nonetheless, your advice is useful. I have discussed this with a few peers in my psych. department and it's coming down to ensuring that both a non-para and para equivalent is used on the data, and if both produce the same result in terms of significance, then we'd report the para result. That would hopefully be defendable in a viva situation. Yet, even this to me seems rather 'unscientific', but since most are using parametric tests, I'll go with the tide. Thanks, J -
Hey everyone, I have a controversial question about the use of statistics in our field. The 3 parametric criteria (i.e., interval level of measurement of above, homogeneity of variance, normal distribution) - how strict do people adhere to these? As you might have noticed, there is a much greater number of studies (certainly in I/O psychology) that just stick to parametric stats, and that puzzles me. I've read that parametric tests are quite 'robust' in that there can be violations in the data in terms of the 3 criteria. If this is the case, does it matter if one chooses to use parametric even if the data violates the criteria? If a non-para test produces a significant results, and it's para equivalent does too, should i just report the latter? Obviously, those from the old school would argue for the strict adherence to the 3 criteria, but i'm suspecting so many people don't! I'd prefer to use para as for some implicit reason it seems more impressive (stupid reason I know, but surely i'm not the only one). So how do you feel about this issue? Please advise. Thanks Jon
-
Hi everyone, another statistical question: If 0= no correlation, 1=perfect correlation, then how can a 'r' statistic be significant (p < .05) when it's stands at .14 for instance? (that's what my spss is reporting). Since this amounts to only 1% of the variance of one variable being accounted for by the variance of the other variable, this really does perplex me. Can someone give some guidance on this? Many thanks, Jon
-
Effect Size of a correlation
nonstoptaxi replied to nonstoptaxi's topic in Psychiatry and Psychology
thanks glider/aommaster, that's most helpful. I've come across r squared before to show the variation accounted for, so i'll use that in my reporting. Cheers! J -
Hi everyone, need advice on this. I realise that it should now be best practice to report effect sizes with respects to reporting on statistical results. I'm fine with this on anovas, t-tests, etc. However, I'm having trouble knowing if i should be reporting (or even if it is possible/appropriate) an effect size for a correlation (specifically a pearson's). Can someone give guidance on this? If I do have to do this, can you please give me the formula to calculate it correlation? Many thanks, await impatiently for some help. Cheers. J